While AI offers significant benefits in transforming production scheduling, its implementation also comes with a set of challenges that manufacturers need to navigate. Here are some of the key challenges of using AI in production scheduling
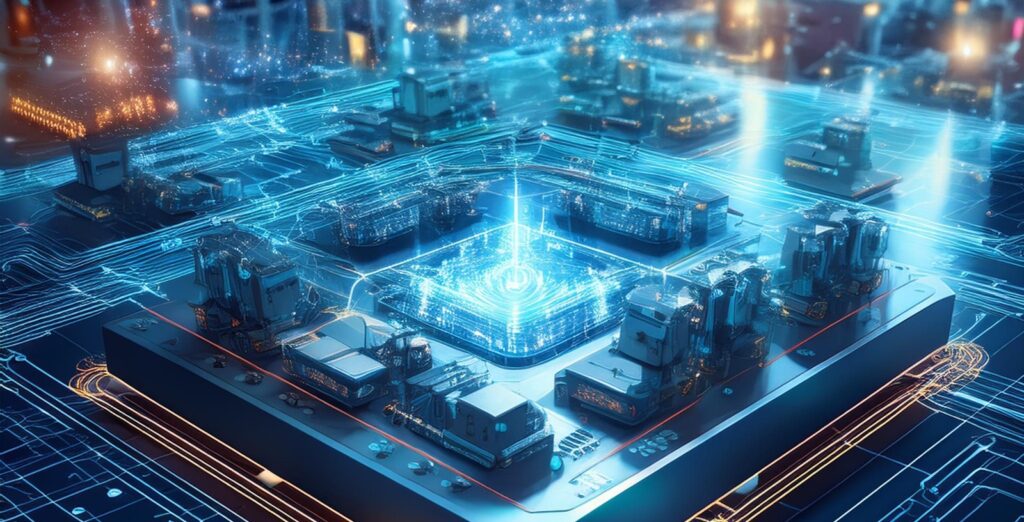
Data Quality and Availability |
|
Challenge: AI algorithms require large volumes of high-quality data to function effectively. Inconsistent, incomplete, or inaccurate data can lead to suboptimal scheduling decisions |
Solution: Implement robust data management practices, including regular data cleaning, validation, and integration of data from various sources like IoT devices, ERP systems, and legacy systems. |
Complexity of AI Models |
|
Challenge: The complexity of AI models can make them difficult to understand and manage, especially for teams without deep technical expertise in AI. This can lead to resistance from stakeholders who are uncomfortable relying on “black box” solutions |
Solution: Invest in training and upskilling employees to build AI literacy. Use explainable AI (XAI) techniques to make AI models more transparent and understandable to non-experts |
Integration with Existing Systems |
|
Challenge: Integrating AI-driven scheduling solutions with existing legacy systems, ERP software, and other IT infrastructure can be challenging, especially if these systems are not designed to handle the complexities of AI. |
Solution: Conduct a thorough assessment of current systems and gradually integrate AI solutions, ensuring compatibility and minimizing disruptions. Consider using middleware or API-driven integration approaches to bridge gaps between old and new technologies. |
Scalability Issues |
|
Challenge: AI models that work well in a pilot or small-scale environment might struggle to maintain performance and accuracy when scaled up to handle the complexities of full production operations |
Solution: Start with scalable AI solutions that can grow with the business. Continuously monitor and refine AI models as they scale, ensuring they can handle increased data volume and complexity. |
Cost and Resource Constraints |
|
Challenge: Implementing AI in production scheduling can require significant upfront investment in technology, infrastructure, and skilled personnel. This can be a barrier for small to medium-sized enterprises (SMEs) with limited resources |
Solution: Explore cost-effective AI solutions, such as cloud-based AI services, that reduce the need for heavy initial investments. Consider phased implementation to spread costs over time and demonstrate ROI before further investment. |
Change Management and Workforce Resistance |
|
Challenge: AI-driven production scheduling may face resistance from the workforce, especially from those accustomed to traditional methods. There may be fears about job displacement or mistrust of AI-generated decisions |
Solution: Implement a comprehensive change management strategy that includes clear communication about the benefits of AI, training programs to ease the transition, and involvement of employees in the AI implementation process |
Ethical and Compliance Concerns |
|
Challenge: The use of AI in production scheduling may raise ethical concerns, particularly around data privacy, algorithmic bias, and compliance with industry regulations. |
Solution: Develop AI governance frameworks that address ethical concerns, ensure compliance with relevant regulations, and promote transparency in AI decision-making processes. |
Adapting to Unforeseen Events |
|
Challenge: While AI can handle many variables, it may struggle to adapt to completely unforeseen events, such as sudden geopolitical shifts, natural disasters, or other black swan events that disrupt the supply chain or production process |
Solution: Complement AI-driven scheduling with robust contingency planning and human oversight to quickly adapt to unexpected disruptions |
Security Risks |
|
Challenge: AI systems, especially those integrated with IoT and other connected devices, can be vulnerable to cyber-attacks, which could compromise production schedules and lead to significant operational disruptions |
Solution: Implement strong cybersecurity measures, including encryption, regular security audits, and real-time monitoring, to protect AI systems from potential threats |
Uncertain ROI |
|
Challenge: Measuring the return on investment (ROI) from AI implementation in production scheduling can be difficult, especially in the early stages. There may be scepticism about whether AI will deliver the expected benefits |
Solution: Set clear, measurable goals for AI implementation and track performance metrics closely. Use pilot projects to demonstrate ROI before scaling up, and ensure that expectations are aligned across the organization |
These challenges highlight the importance of a strategic and thoughtful approach when implementing AI in production scheduling. By addressing these hurdles proactively, manufacturers can better position themselves to reap the benefits of AI while minimizing risks.